Dacon 대회의 코드 공유를 보면서 Plotly를 알 수 있었고 동적인 그래프인데, 몇 줄 안되는 코드로 구현되는 것이 정말 신기했고 기초부터 조금씩 알아보자는 의도로 글을 작성하게 되었다. plotly 공식 홈페이지에서 예제들을 보고 정리한 글이다. Plotly 패키지는 Interactive한 그래프로 동적인 그래프를 만들 수 있다.
기본적인 사용 방식은 아래와 같다.
from plotly.subplots import make_subplots
import plotly.graph_objects as go
fig = make_subplots(rows = n, cols = r) # 행과 열의 숫자를 지정해주면 원하는 axes만큼의 그래프가 생성된다.
fig.add_trace(go.Scatter(x = [1,2,3], y = [4,5,6],
row = n, col = r) # (n,r) axes에 그래프가 생성된다.
fig.update_layout(height = 600, width = 800, title_text = "Side by Side Subplots") # title을 지정해줄 수 있다.
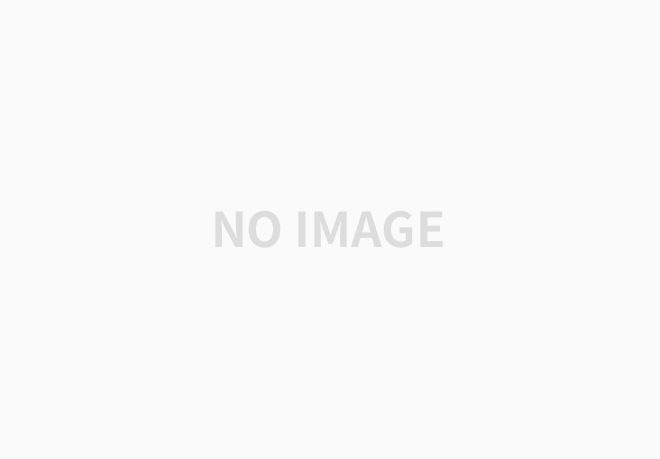
원하는 axes의 수를 설정하여 fig 변수에 지정해주었다. 이후 barplot, scatter plot 등 원하는 그림을 plotly.graph_objects 라이브러리를 활용하여 그리면 되고 axes도 지정해주어야 한다. fig.update_layout은 그림의 너비, 길이, 제목 등을 조정해주는 메서드이다.
현재, 사진을 가져왔을 때에는 정적인 그래프처럼 보이지만, Jupyter notebook에서 실행했을 경우, 각 점에 마우스 포인터를 가져다 놓는다면, 해당 점의 x축과 y축도 함께 보여준다.
Plotly의 graph_objects 라이브러리 말고도 Plotly.express 라이브러리를 활용하여 보다 쉽고 간결하게 그래프를 그릴 수 있다. 검색해본 결과, Plotly와 Plotly.express의 차이점은 Plotly에서는 'hue' (그룹을 나누어주는) 인자가 없지만, Plotly.express에는 'hue' 와 'color' 등의 인자가 존재해, Plotly를 보다 쉽게 활용할 수 있게 해준다.
iris 데이터를 예제로 Plotly.express를 구현해보자.
# irir 데이터 불러오기
import pandas as pd
import numpy as np
from sklearn.datasets import load_iris
iris = load_iris()
df_iris = pd.DataFrame(data=iris.data, columns=iris.feature_names)
df_iris['target'] = iris.target
df_iris['target'] = df_iris['target'].map({0:"setosa", 1:"versicolor", 2:"virginica"})
# plotly.graph_object로 구현한 경우
fig = make_subplots(rows=2, cols=2)
fig.add_trace(go.Bar(x = df_iris['sepal length (cm)'], y = df_iris['sepal width (cm)']), row = 1, col = 1)
fig.update_layout(width = 600, height = 600)
fig.show()
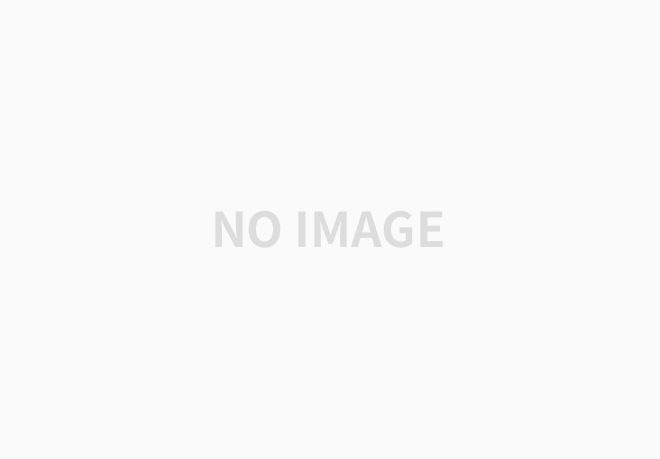
# plotly.express로 구현한 경우
fig = px.scatter(df_iris, x = "sepal length (cm)", y = "sepal width (cm)", color = 'target', hover_data = ['target'])
fig.update_layout(height = 600, width = 600, hovermode = 'closest', title_text = "sepal length vs sepal width")
fig.show()
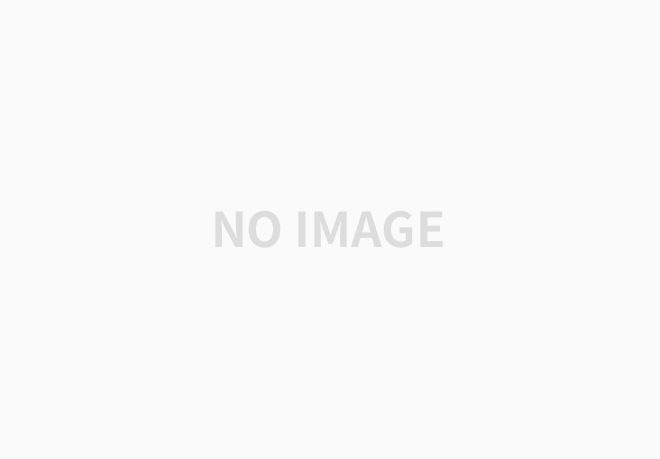
plotly와 plotly.express의 코드를 본다면, plotly.express는 color에 'target' 구분자를 주어 구분할 수 있지만, plotly의 경우 express와 같은 그래프로 나타내려면 add_trace 인자에 marker와 color를 추가해주어야 한다.
*hover_data : 마우스 포인터를 가져다 놓았을 떄, 보고 싶은 변수의 값을 추가로 보여준다.
plotly.express의 인자인 marginal_x는 x축 변수에 해당하는 데이터의 분포를 main plot 위에 보여주고 marginal_y는 y축 변수에 해당하는 데이터의 분포를 main plot 오른쪽에 보여준다.
fig = px.scatter(df_iris, x = "sepal length (cm)", y = "sepal width (cm)", color = 'target', hover_data = ['target'],\
marginal_x = 'box', marginal_y = 'box')
fig.update_layout(height = 600, width = 600, hovermode = 'closest', title_text = "sepal length vs sepal width")
fig.show()
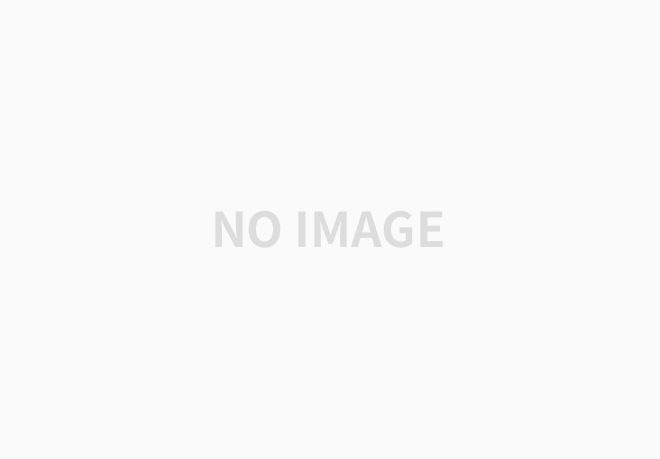
오늘 공부한 Plotly는 여기까지다. 계속해서 Python을 사용하면서 모르는 것이 있다면 추가해야지🙆♂️
'Algorithm > Python' 카테고리의 다른 글
[백준 11399번 - ATM] (0) | 2023.01.24 |
---|---|
[백준 2720번 - 세탁소 사장 동혁] (0) | 2023.01.24 |
[Python] Optuna 사용법 (0) | 2021.07.07 |
[Python] Matplotlib 그림의 제목 조절 방법 (0) | 2021.02.03 |
[Python] Json, Request (2) | 2021.01.14 |